Clinical Data Warehouse
Working shoulder-to-shoulder, a team of physicians, systems biologists, engineers, and scientists at Rutgers Cancer Institute of New Jersey (RCINJ) have designed, developed, and implemented the Warehouse with information originating from data sources, including Electronic Medical Records, Clinical Trial Management Systems, Tumor Registries, Biospecimen Repositories, Radiology and Pathology imaging archives, and Next Generation Sequencing services. Innovative solutions were implemented to detect and extract unstructured clinical information that was embedded in paper/text documents, including synoptic pathology reports. RCINJ is one of the first Institutions to implement a multi-modal oncology-based Clinical Data Warehouse.
The growing system enables physicians to systematically mine and review the molecular, genomic, image-based, and correlated clinical information of patient tumors individually or as part of large cohorts to identify changes and patterns that may influence treatment decisions and potential outcomes. An Informatica-based (Redwood City, CA) extraction transformation and load (ETL) interface automatically populates the secure, IRB-approved, Oracle-based (Redwood Shores, CA) Warehouse with data elements originating from each of the multi-modal data sources. The ETL for the CRDW supports the dynamic expansion of the C&RDW with a range of multimodal data (Figure 1). In May 2020, Dr. Foran’s team collaborated with the Google Healthcare division to successfully migrate an instance of the Warehouse onto the Google Cloud Platform (GCP) and demonstrated its functionality at scale as part of a proof-of-concept pilot.
Based on the experience gained by the team during this project, a decision was made to continue to utilize the on-premises version of the ETL while continuing to develop cloud-based solutions for the CRDW going forward.
The co-localization of such a broad number of correlated data elements representing the full spectrum of clinical information, imaging studies and genomic information coupled with our experience and expertise in advanced pattern recognition, high-performance computing and data mining has positioned our team with unique opportunities to optimize personalizing treatment, refine best practices and provide objective, reproducible insight as to the underlying mechanisms of disease onset and progression.
This effort has recently been elevated to an enterprise-wide Clinical & Research Data Warehouse (CRDW) project that now encompasses both oncology and non-oncology areas of medicine. A close collaboration between the original developers at Rutgers Cancer Institute of NJ, Rutgers Office of Information Technology (OIT) and Rutgers Office of Information Technology and the Office of Advanced Research Computing (OARC) has led to the construction of a secure environment to support state-of-the-art AI & Machine-Learning pipelines with ready access to high-performance and cloud computing. The platform facilitates reliable integration and analysis of sensitive data. Using these assets, our team hopes to accelerate the pace of developing these methods and algorithms to support a wide range of clinical applications and translational research projects.
Some examples of successful projects reliant upon CRDW:
Our team modified ETL and data model of CRDW to support two projects with the overarching objective to establish a COVID-19 data registry of patients across a consortium of institutions including diagnoses, risk factors, clinical findings and outcomes –
Project 1 – COMBATCOVID: Consortium for Multisite Biomedical Analytics and Trials on COVID-19. (NYU, Buffalo, Einstein, Icahn, UPenn)
Project 2 – The National COVID Cohort Collaborative (N3C): Partnership among CTSA Program hubs, the National Center for Data to Health (CD2H), with oversight by NCATS.
The Warehouse is made available to investigators throughout the clinical and research communities through a Clinical and Translational Science Award (CTSA) called the New Jersey Alliance for Clinical and Translational Science (7UL1TR003017-05). NJ ACTS comprises a consortium with Rutgers and Princeton Universities (PU), NJ Institute for Technology (NJIT), medical, nursing, dental and public health schools, hospitals, community health centers, outpatient practices, industry, policymakers and health information exchanges. All Alliance universities and affiliates have provided substantial resources and contributed to the planning, development and leadership of the consortium. With access to ~7 million people, NJ ACTS serves as a ‘natural laboratory’ for translational and clinical research. With a state population of ~9 million, New Jersey ranks 11th in the US, 1st in population density and higher than average in racial and ethnic diversity. Our
CTSA Hub focuses on two overarching themes: the heterogeneity of disease pathogenesis and response to treatment, and the value of linking large clinical databases with interventional clinical investigations to identify cause-and-effect and predict therapeutic responses. NJ ACTS provides: innovative approaches to link information from large databases and electronic health records to inform clinical trial design, execution and analysis; and novel platforms for biomarker discovery using fluorescence in situ hybridization and machine learning to identify unique neural signatures of chronic illness. NJ ACTS will access a large health system with significant member diversity; a rich legacy of community engagement and community-based research platforms; and proven approaches to enhance workforce development in clinical research.
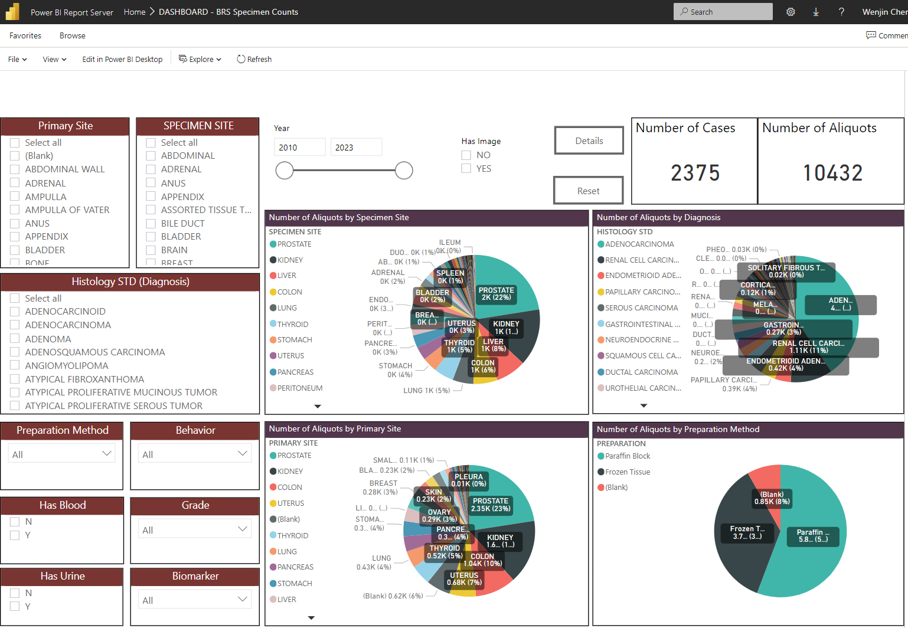
Working closely with the faculty and staff at Biospecimens Repository Shared Resource at CINJ, the Warehouse effectively standardized pathology data associated with banked tissue specimen to enable a graphical dashboard that can be easily queried by researchers. By further associating case information with staging information as well as deidentified whole slide images, the dashboard help the Director and staff to effectively help resource users forming study cohorts and provide specimens.
The CRDW is also one of the primary sources for digital pathology and clinical correlates for a dynamic pathology quiz bank that will be used to training medical students and residents at University of Botswana and RWJBH that features virtual telemicrosocpy, automated scoring and the growing database of oncology cases
As we develop workflows to support the wider Rutgers research community, the Warehouse team has developed a Dashboard interface for the CRDW to facilitate initiation of queries of de-identified data within the Warehouse by enabling investigators to assess the feasibility of conducting specific research studies.
As part of an NCI-funded project (UH3-CA225021) our team is collaborating with investigators at Stony Brook University and cancer registries in Georgia, Kentucky, New Jersey and New York to enrich Surveillance, Epidemiology, and End Results (SEER) registry data with high‐quality population‐based biospecimen data in the form of digital pathology, machine learning based classifications and quantitative pathomics feature sets. To facilitate this work, the Warehouse serves as a local repository for data collection. Our team has already begun to investigate the use of neural networks to automate the analysis of digitized pathology specimens. For example, this figure shows the feature map representation of TIL and tumor analysis of digitized TCGA BRCA specimen based on VGG16 and ResNet neural networks. Upper right corner: tumor segmentation map. Lower left corner: the TIL map. Lower right corner: combined and thresholded TIL and tumor maps.
A few relevant publications:
1. Foran, DJ et al. Roadmap to a Comprehensive Clinical Data Warehouse for Precision Medicine Applications in Oncology. Cancer Informatics. 2017. PMCID: PMC5392017
2. Ren J, Karagoz K, Gatza ML, Singer EA, Sadimin E, Foran DJ, Qi X. Recurrence analysis on prostate cancer patients with Gleason score 7 using integrated histopathology whole-slide images and genomic data through deep neural networks. J Med Imaging. 2018. PMID: 30840742; PMCID: PMC6237203.
3. Ren J, Singer EA, Sadimin E, Foran DJ, Qi X. Statistical Analysis of Survival Models using Feature Quantification on Prostate Cancer Histopathology Images. Journal of Pathology Informatics. 2019. PMCID: PMC6788183
4. Qi X, Brown L, Foran DJ, Nosher J, Hacihaliloglu I. Chest X-ray image phase features for improved diagnosis of COVID-19 using convolutional neural networks. International Journal of Computer Assisted Radiology and Surgery. 2021 January; doi: 10.1007/s11548-020-02305-w.
5. David J. Foran, Eric B. Durbin,, Wenjin Chen, Evita Sadimin, Ashish Sharma,Imon Banerjee, Tahsin Kurc, Nan Li, Antoinette M. Stroup, Gerald Harris, Annie Gu, Maria Schymura , Rajarsi Gupta, Erich Bremer, Joseph Balsamo, Tammy DiPrima, Feiqiao Wang, Shahira Abousamra, Dimitris Samaras, Isaac Hands, Kevin Ward and Joel H. Saltz. An Expandable Informatics Framework for Enhancing Central Cancer Registries with Digital Pathology Specimens, Computational Imaging Tools & Advanced Mining Capabilities. J Pathol Inform 2022;13:5, 1-11. Available FREE in open access from: http://www.jpathinformatics.org/text.asp?2022/13/1/5/334787.
6. David J. Foran, Nhan Do, Samuel Ajjarapu, Wenjin Chen, Tahsin Kurc, Joel H. Saltz. An Intelligent Search and Retrieval System for Mining Clinical Data Repositories Based on Computational Imaging Markers and Genomic Expression Signatures for Investigative Research and Decision Support. International Scholarly and Scientific Research & Innovation 17(09) 2023.
7. David J. Foran, Wenjin Chen, Tahsin Kurc, Antoinette M. Stroup, Gerald Harris, Rajarsi Gupta, Erich Bremer, Jakub Kaczmarzyk, Luke Torre-Healy, Samuel Ajjarapu, Nhan Do, Eric B. Durbin,,, Kevin Ward and Joel H. Saltz. An Intelligent Search & Retrieval System (IRIS) and Clinical and Research Repository for Decision Support based on Machine Learning and Joint Kernel-based Supervised Hashing. Cancer Inform. 2024 Feb 4;23:11769351231223806. doi: 10.1177/11769351231223806. PMID: 38322427; PMCID: PMC10840403.